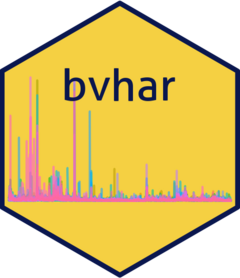
Fitting Bayesian VHAR of Minnesota Prior
Source:R/bvhar-minnesota.R
, R/print-bvharmn.R
, R/criteria.R
, and 1 more
bvhar_minnesota.Rd
This function fits BVHAR with Minnesota prior.
Usage
bvhar_minnesota(
y,
har = c(5, 22),
num_chains = 1,
num_iter = 1000,
num_burn = floor(num_iter/2),
thinning = 1,
bayes_spec = set_bvhar(),
scale_variance = 0.05,
include_mean = TRUE,
parallel = list(),
verbose = FALSE,
num_thread = 1
)
# S3 method for class 'bvharmn'
print(x, digits = max(3L, getOption("digits") - 3L), ...)
# S3 method for class 'bvharhm'
print(x, digits = max(3L, getOption("digits") - 3L), ...)
# S3 method for class 'bvharmn'
logLik(object, ...)
# S3 method for class 'bvharmn'
AIC(object, ...)
# S3 method for class 'bvharmn'
BIC(object, ...)
is.bvharmn(x)
# S3 method for class 'bvharmn'
knit_print(x, ...)
# S3 method for class 'bvharhm'
knit_print(x, ...)
Arguments
- y
Time series data of which columns indicate the variables
- har
Numeric vector for weekly and monthly order. By default,
c(5, 22)
.- num_chains
Number of MCMC chains
- num_iter
MCMC iteration number
- num_burn
Number of burn-in (warm-up). Half of the iteration is the default choice.
- thinning
Thinning every thinning-th iteration
- bayes_spec
A BVHAR model specification by
set_bvhar()
(default) orset_weight_bvhar()
.- scale_variance
Proposal distribution scaling constant to adjust an acceptance rate
- include_mean
Add constant term (Default:
TRUE
) or not (FALSE
)- parallel
List the same argument of
optimParallel::optimParallel()
. By default, this is empty, and the function does not execute parallel computation.- verbose
Print the progress bar in the console. By default,
FALSE
.- num_thread
Number of threads
- x
Any object
- digits
digit option to print
- ...
not used
- object
A
bvharmn
object
Value
bvhar_minnesota()
returns an object bvharmn
class.
It is a list with the following components:
- coefficients
Posterior Mean
- fitted.values
Fitted values
- residuals
Residuals
- mn_mean
Posterior mean matrix of Matrix Normal distribution
- mn_prec
Posterior precision matrix of Matrix Normal distribution
- iw_scale
Posterior scale matrix of posterior inverse-wishart distribution
- iw_shape
Posterior shape of inverse-Wishart distribution (\(\nu_0\) - obs + 2). \(\nu_0\): nrow(Dummy observation) - k
- df
Numer of Coefficients: 3m + 1 or 3m
- m
Dimension of the time series
- obs
Sample size used when training =
totobs
- 22- prior_mean
Prior mean matrix of Matrix Normal distribution: \(M_0\)
- prior_precision
Prior precision matrix of Matrix Normal distribution: \(\Omega_0^{-1}\)
- prior_scale
Prior scale matrix of inverse-Wishart distribution: \(\Psi_0\)
- prior_shape
Prior shape of inverse-Wishart distribution: \(\nu_0\)
- y0
\(Y_0\)
- design
\(X_0\)
- p
3, this element exists to run the other functions
- week
Order for weekly term
- month
Order for monthly term
- totobs
Total number of the observation
- type
include constant term (
const
) or not (none
)- HARtrans
VHAR linear transformation matrix: \(C_{HAR}\)
- y
Raw input (
matrix
)- call
Matched call
- process
Process string in the
bayes_spec
:BVHAR_MN_VAR
(BVHAR-S) orBVHAR_MN_VHAR
(BVHAR-L)- spec
Model specification (
bvharspec
)
It is also normaliw
and bvharmod
class.
Details
Apply Minnesota prior to Vector HAR: \(\Phi\) (VHAR matrices) and \(\Sigma_e\) (residual covariance).
$$\Phi \mid \Sigma_e \sim MN(M_0, \Omega_0, \Sigma_e)$$ $$\Sigma_e \sim IW(\Psi_0, \nu_0)$$ (MN: matrix normal, IW: inverse-wishart)
There are two types of Minnesota priors for BVHAR:
VAR-type Minnesota prior specified by
set_bvhar()
, so-called BVHAR-S model.VHAR-type Minnesota prior specified by
set_weight_bvhar()
, so-called BVHAR-L model.
References
Kim, Y. G., and Baek, C. (2024). Bayesian vector heterogeneous autoregressive modeling. Journal of Statistical Computation and Simulation, 94(6), 1139-1157.
See also
set_bvhar()
to specify the hyperparameters of BVHAR-Sset_weight_bvhar()
to specify the hyperparameters of BVHAR-Lsummary.normaliw()
to summarize BVHAR model
Examples
# Perform the function using etf_vix dataset
fit <- bvhar_minnesota(y = etf_vix[,1:3])
class(fit)
#> [1] "bvharmn" "bvharmod" "normaliw"
# Extract coef, fitted values, and residuals
coef(fit)
#> GVZCLS OVXCLS VXFXICLS
#> GVZCLS_day 9.563078e-01 0.0049571622 0.0023012975
#> OVXCLS_day -1.124095e-03 0.9934581488 0.0021059646
#> VXFXICLS_day -3.655575e-04 -0.0196286064 0.9707085757
#> GVZCLS_week 7.114698e-04 0.0003115497 -0.0029533997
#> OVXCLS_week -1.272095e-03 -0.0007333494 -0.0010949289
#> VXFXICLS_week 2.842160e-03 0.0074516208 -0.0054726494
#> GVZCLS_month 9.807968e-03 0.0035140103 0.0030645736
#> OVXCLS_month -8.954785e-05 0.0008878183 0.0008366598
#> VXFXICLS_month 1.183747e-03 0.0012979345 -0.0008406757
#> const 5.747434e-01 0.2878285856 0.7849725826
head(residuals(fit))
#> [,1] [,2] [,3]
#> [1,] 0.31184618 -0.01743843 -0.26993926
#> [2,] -0.57825789 0.17050304 -0.64147554
#> [3,] -0.24663283 0.44836077 3.18688370
#> [4,] -0.61879508 0.57710447 -1.03884178
#> [5,] 0.18365992 0.42795339 -0.09427081
#> [6,] 0.06251278 1.29400222 0.49667649
head(fitted(fit))
#> GVZCLS OVXCLS VXFXICLS
#> [1,] 20.37815 32.33744 29.72994
#> [2,] 20.60826 32.25950 29.31148
#> [3,] 19.97663 32.38164 28.54312
#> [4,] 19.68880 32.72290 31.50884
#> [5,] 19.05634 33.21205 30.28427
#> [6,] 19.21749 33.55600 30.01332