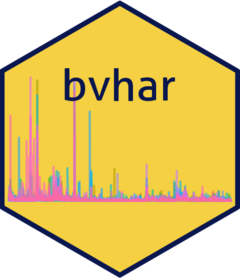
Fitting Vector Heterogeneous Autoregressive Model
Source:R/vharlse.R
, R/print-vharlse.R
, R/criteria.R
, and 1 more
vhar_lm.Rd
This function fits VHAR using OLS method.
Usage
vhar_lm(
y,
har = c(5, 22),
include_mean = TRUE,
method = c("nor", "chol", "qr")
)
# S3 method for class 'vharlse'
print(x, digits = max(3L, getOption("digits") - 3L), ...)
# S3 method for class 'vharlse'
logLik(object, ...)
# S3 method for class 'vharlse'
AIC(object, ...)
# S3 method for class 'vharlse'
BIC(object, ...)
is.vharlse(x)
# S3 method for class 'vharlse'
knit_print(x, ...)
Arguments
- y
Time series data of which columns indicate the variables
- har
Numeric vector for weekly and monthly order. By default,
c(5, 22)
.- include_mean
Add constant term (Default:
TRUE
) or not (FALSE
)- method
Method to solve linear equation system. (
nor
: normal equation (default),chol
: Cholesky, andqr
: HouseholderQR)- x
Any object
- digits
digit option to print
- ...
not used
- object
A
vharlse
object
Value
vhar_lm()
returns an object named vharlse
class.
It is a list with the following components:
- coefficients
Coefficient Matrix
- fitted.values
Fitted response values
- residuals
Residuals
- covmat
LS estimate for covariance matrix
- df
Numer of Coefficients
- m
Dimension of the data
- obs
Sample size used when training =
totobs
-month
- y0
Multivariate response matrix
- p
3 (The number of terms.
vharlse
contains this element for usage in other functions.)- week
Order for weekly term
- month
Order for monthly term
- totobs
Total number of the observation
- process
Process: VHAR
- type
include constant term (
const
) or not (none
)- HARtrans
VHAR linear transformation matrix
- design
Design matrix of VAR(
month
)- y
Raw input
- method
Solving method
- call
Matched call
It is also a bvharmod
class.
Details
For VHAR model
$$Y_{t} = \Phi^{(d)} Y_{t - 1} + \Phi^{(w)} Y_{t - 1}^{(w)} + \Phi^{(m)} Y_{t - 1}^{(m)} + \epsilon_t$$
the function gives basic values.
References
Baek, C. and Park, M. (2021). Sparse vector heterogeneous autoregressive modeling for realized volatility. J. Korean Stat. Soc. 50, 495-510.
Bubák, V., Kočenda, E., & Žikeš, F. (2011). Volatility transmission in emerging European foreign exchange markets. Journal of Banking & Finance, 35(11), 2829-2841.
Corsi, F. (2008). A Simple Approximate Long-Memory Model of Realized Volatility. Journal of Financial Econometrics, 7(2), 174-196.
See also
summary.vharlse()
to summarize VHAR model
Examples
# Perform the function using etf_vix dataset
fit <- vhar_lm(y = etf_vix)
class(fit)
#> [1] "vharlse" "olsmod" "bvharmod"
str(fit)
#> List of 19
#> $ coefficients : num [1:28, 1:9] 0.8698 0.02988 -0.01632 -0.10078 0.00306 ...
#> ..- attr(*, "dimnames")=List of 2
#> .. ..$ : chr [1:28] "GVZCLS_day" "OVXCLS_day" "VXFXICLS_day" "VXEEMCLS_day" ...
#> .. ..$ : chr [1:9] "GVZCLS" "OVXCLS" "VXFXICLS" "VXEEMCLS" ...
#> $ fitted.values: num [1:883, 1:9] 20.4 20.6 20.1 19.9 19.3 ...
#> $ residuals : num [1:883, 1:9] 0.3176 -0.5873 -0.3752 -0.8374 -0.0281 ...
#> $ covmat : num [1:9, 1:9] 1.119 0.375 0.299 0.437 1.37 ...
#> ..- attr(*, "dimnames")=List of 2
#> .. ..$ : chr [1:9] "GVZCLS" "OVXCLS" "VXFXICLS" "VXEEMCLS" ...
#> .. ..$ : chr [1:9] "GVZCLS" "OVXCLS" "VXFXICLS" "VXEEMCLS" ...
#> $ df : int 28
#> $ m : int 9
#> $ obs : int 883
#> $ y0 : num [1:883, 1:9] 20.7 20 19.7 19.1 19.2 ...
#> ..- attr(*, "dimnames")=List of 2
#> .. ..$ : NULL
#> .. ..$ : chr [1:9] "GVZCLS" "OVXCLS" "VXFXICLS" "VXEEMCLS" ...
#> $ p : int 3
#> $ week : int 5
#> $ month : int 22
#> $ totobs : num 905
#> $ process : chr "VHAR"
#> $ type : chr "const"
#> $ HARtrans : num [1:28, 1:199] 1 0 0 0 0 0 0 0 0 0.2 ...
#> $ design : num [1:883, 1:199] 20.4 20.7 20 19.7 19.1 ...
#> $ y : num [1:905, 1:9] 21.5 21.5 22.3 21.6 21.2 ...
#> ..- attr(*, "dimnames")=List of 2
#> .. ..$ : NULL
#> .. ..$ : chr [1:9] "GVZCLS" "OVXCLS" "VXFXICLS" "VXEEMCLS" ...
#> $ method : chr "nor"
#> $ call : language vhar_lm(y = etf_vix)
#> - attr(*, "class")= chr [1:3] "vharlse" "olsmod" "bvharmod"
# Extract coef, fitted values, and residuals
coef(fit)
#> GVZCLS OVXCLS VXFXICLS VXEEMCLS
#> GVZCLS_day 0.869796005 -0.04681230 -0.0172897224 0.049002224
#> OVXCLS_day 0.029877159 0.91358257 0.0350799026 0.010047707
#> VXFXICLS_day -0.016319909 -0.13959548 0.8575034005 -0.033709406
#> VXEEMCLS_day -0.100780743 -0.08210474 0.0121290737 0.655662834
#> VXSLVCLS_day 0.003060357 0.03668387 0.0282158214 0.013830263
#> EVZCLS_day 0.186426595 -0.17282244 0.0494563262 -0.062064078
#> VXXLECLS_day 0.078818472 0.10515479 0.0728823779 0.279901357
#> VXGDXCLS_day -0.039080303 0.04925811 -0.0428945531 -0.033003536
#> VXEWZCLS_day 0.032080106 0.07853175 0.0131343314 0.069517084
#> GVZCLS_week -0.040418408 -0.07188231 -0.0938952991 -0.157648897
#> OVXCLS_week -0.085524289 -0.06406862 -0.0701989801 -0.038293713
#> VXFXICLS_week -0.029907441 0.12562086 0.0749857892 0.139908054
#> VXEEMCLS_week 0.230258633 0.05761231 0.0006402918 0.126209971
#> VXSLVCLS_week 0.027500102 0.02993123 0.0442818765 0.042748861
#> EVZCLS_week -0.236308396 0.28602174 -0.0005685013 0.140233072
#> VXXLECLS_week -0.120360477 -0.05095917 -0.1126391194 -0.332190330
#> VXGDXCLS_week 0.054947386 -0.06808223 0.0506682953 0.025319297
#> VXEWZCLS_week -0.043554254 -0.05055300 0.0078698375 -0.007277838
#> GVZCLS_month 0.023458944 0.01754305 -0.0253911426 0.003596504
#> OVXCLS_month 0.001541434 0.07383772 -0.0059125573 -0.012132076
#> VXFXICLS_month -0.011772882 -0.02021036 -0.0587438676 -0.128208430
#> VXEEMCLS_month -0.134015581 -0.03842329 -0.0059768395 0.135807907
#> VXSLVCLS_month -0.002659358 -0.01415388 -0.0158012078 0.038533007
#> EVZCLS_month 0.185251113 -0.05458701 0.1512782039 -0.012040779
#> VXXLECLS_month 0.105997340 0.09819774 0.0508482758 0.101087018
#> VXGDXCLS_month 0.033825430 0.05583194 0.0377451676 0.024847951
#> VXEWZCLS_month 0.020348604 -0.01547358 -0.0074385193 -0.065668936
#> const 0.257508640 -0.75244225 0.7874223172 0.329901821
#> VXSLVCLS EVZCLS VXXLECLS VXGDXCLS
#> GVZCLS_day 0.2171686933 -8.528593e-04 -0.006316449 0.14468642
#> OVXCLS_day -0.0038224358 1.317614e-02 0.023484352 0.06339221
#> VXFXICLS_day -0.0821022074 -2.145593e-02 -0.074931174 -0.05632922
#> VXEEMCLS_day -0.0951773758 -1.278904e-02 -0.111052628 -0.05281887
#> VXSLVCLS_day 0.6911996371 4.988352e-03 0.009943586 0.07416401
#> EVZCLS_day 0.2247289953 8.908482e-01 -0.164489289 -0.02651442
#> VXXLECLS_day 0.1848839755 2.688135e-02 1.110417850 0.20178781
#> VXGDXCLS_day -0.0326865958 -1.097720e-02 0.015583137 0.65845387
#> VXEWZCLS_day 0.0086576066 2.741950e-02 0.057451312 0.06060228
#> GVZCLS_week -0.1717505332 5.820058e-03 -0.062671994 -0.07765708
#> OVXCLS_week 0.0013998157 -3.043820e-02 -0.081955633 -0.06014147
#> VXFXICLS_week 0.0351147921 4.615955e-02 0.148110845 0.14285833
#> VXEEMCLS_week 0.2351233848 5.680828e-03 0.007968589 0.02454380
#> VXSLVCLS_week 0.1788828526 -8.453363e-05 0.023363480 -0.06368503
#> EVZCLS_week -0.4461793985 1.019828e-02 0.182684876 -0.09790030
#> VXXLECLS_week -0.2019441922 -2.813833e-02 -0.236461970 -0.21181825
#> VXGDXCLS_week 0.0003105573 -7.379189e-03 -0.011074423 0.20755785
#> VXEWZCLS_week -0.0240538695 -2.495159e-02 0.001237576 -0.08368461
#> GVZCLS_month -0.0834085916 -2.856799e-02 -0.047705620 -0.12266095
#> OVXCLS_month -0.0657907568 1.107681e-02 0.017294294 -0.05920651
#> VXFXICLS_month -0.1051804654 -1.719717e-02 -0.087376926 -0.15744156
#> VXEEMCLS_month -0.0829087351 -1.492401e-02 0.038494367 -0.02320949
#> VXSLVCLS_month 0.0325418895 1.020723e-02 0.048603609 0.05950528
#> EVZCLS_month 0.5392049317 7.115211e-02 0.002772821 0.24304619
#> VXXLECLS_month 0.0824108862 2.585456e-02 0.176619398 0.06498108
#> VXGDXCLS_month 0.1042356427 2.218512e-02 0.016276608 0.11878639
#> VXEWZCLS_month 0.0298234196 -1.661517e-05 -0.049185431 0.06065243
#> const 0.8310348716 -2.382650e-02 0.258939806 0.60556631
#> VXEWZCLS
#> GVZCLS_day 0.060810440
#> OVXCLS_day -0.021328545
#> VXFXICLS_day -0.103241781
#> VXEEMCLS_day -0.050810494
#> VXSLVCLS_day -0.041214628
#> EVZCLS_day -0.044510366
#> VXXLECLS_day 0.286415660
#> VXGDXCLS_day -0.004629846
#> VXEWZCLS_day 0.952152534
#> GVZCLS_week -0.232659033
#> OVXCLS_week 0.029438686
#> VXFXICLS_week 0.233415007
#> VXEEMCLS_week -0.104629279
#> VXSLVCLS_week 0.174714784
#> EVZCLS_week 0.123320940
#> VXXLECLS_week -0.239829609
#> VXGDXCLS_week -0.012408245
#> VXEWZCLS_week 0.018610770
#> GVZCLS_month 0.334911077
#> OVXCLS_month 0.012987518
#> VXFXICLS_month -0.111977668
#> VXEEMCLS_month 0.133305259
#> VXSLVCLS_month -0.181258940
#> EVZCLS_month -0.129656359
#> VXXLECLS_month -0.041980863
#> VXGDXCLS_month -0.027342181
#> VXEWZCLS_month -0.009420411
#> const 1.047643911
head(residuals(fit))
#> [,1] [,2] [,3] [,4] [,5] [,6]
#> [1,] 0.31758364 -0.11022615 -0.5296023 0.04716717 -0.5378020 -0.4659005
#> [2,] -0.58729937 -0.02977095 -0.9556970 -0.70126942 -1.4850409 -0.5500010
#> [3,] -0.37519058 0.15466899 2.8092305 1.54460103 -0.4209523 0.4631326
#> [4,] -0.83743454 0.32276054 -1.2472184 -1.66328320 -2.0118629 -0.4732741
#> [5,] -0.02811876 0.29432487 -0.2286953 0.48869052 0.5149326 0.3606493
#> [6,] -0.14617138 1.20662142 0.3416573 1.34978100 -0.7638851 0.6622565
#> [,7] [,8] [,9]
#> [1,] -0.03896889 -0.8878031 0.8547725
#> [2,] -0.01517932 -1.2314864 -0.6129635
#> [3,] 2.49685115 0.9469600 2.3957692
#> [4,] -2.00237189 -1.4008812 -1.1284324
#> [5,] -0.26141669 0.4395067 1.5021262
#> [6,] 0.71563874 0.7208909 1.7436068
head(fitted(fit))
#> [,1] [,2] [,3] [,4] [,5] [,6] [,7] [,8]
#> [1,] 20.37242 32.43023 29.98960 28.28283 40.30780 12.91590 24.22897 32.12780
#> [2,] 20.61730 32.45977 29.62570 28.64127 39.48504 12.51000 24.56518 32.01149
#> [3,] 20.10519 32.67533 28.92077 28.50540 38.21095 12.07687 25.06315 31.72304
#> [4,] 19.90743 32.97724 31.71722 30.56328 38.13186 12.61327 27.88237 33.30088
#> [5,] 19.26812 33.34568 30.41870 29.20131 36.60507 12.20935 26.08142 32.38049
#> [6,] 19.42617 33.64338 30.16834 29.66022 37.24389 12.58774 25.84436 33.12911
#> [,9]
#> [1,] 29.00523
#> [2,] 29.72296
#> [3,] 29.20423
#> [4,] 31.84843
#> [5,] 30.60787
#> [6,] 31.67639